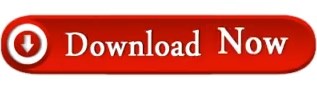

RAM size does not affect deep learning performance. This is so because (1) if you used pinned memory, your mini-batches will be transferred to the GPU without involvement from the CPU, and (2) if you do not use pinned memory the performance gains of fast vs slow RAMs is about 0-3% - spend your money elsewhere! RAM Size This is best explained by “ Does RAM speed REALLY matter?” video on RAM von Linus Tech Tips.įurthermore, it is important to know that RAM speed is pretty much irrelevant for fast CPU RAM->GPU RAM transfers. RAM clock rates are marketing stints where RAM companies lure you into buying “faster” RAM which actually yields little to no performance gains. The second mistake is to buy not enough RAM to have a smooth prototyping experience. The main mistakes with RAM is to buy RAM with a too high clock rate. Suspect line-upĬan you identify the hardware part which is at fault for bad performance? One of these GPUs? Or maybe it is the fault of the CPU after all? RAM Otherwise you might run into temperature issues and your GPUs will be slower (about 30%) and die faster. If you want to stick GPUs into PCIe slots which are next to each other you should make sure that you get GPUs with a blower-style fan. Companies: 8 GB for prototyping, >=11 GB for trainingĪnother problem to watch out for, especially if you buy multiple RTX cards is cooling.Startups: 8 GB (but check the specific application area for model sizes).Research that is hunting for interesting architectures: >=8 GB.Research that is hunting state-of-the-art scores: >=11 GB.In general, the requirements for memory are roughly the following: As such RTX cards have a memory advantage and picking RTX cards and learn how to use 16-bit models effectively will carry you a long way. RTX cards, which can run in 16-bits, can train models which are twice as big with the same memory compared to GTX cards. Otherwise, GTX 1070, GTX 1080, GTX 1070 Ti, and GTX 1080 Ti from eBay are fair choices and you can use these GPUs with 32-bit (but not 16-bit).īe careful about the memory requirements when you pick your GPU. If you use these cards you should use 16-bit models. There are three main mistakes that you can make when choosing a GPU: (1) bad cost/performance, (2) not enough memory, (3) poor cooling.įor good cost/performance, I generally recommend an RTX 2070 or an RTX 2080 Ti. I talked at length about GPU choice in my GPU recommendations blog post, and the choice of your GPU is probably the most critical choice for your deep learning system. The GPU is just the heart of deep learning applications – the improvement in processing speed is just too huge to ignore. If you are building or upgrading your system for deep learning, it is not sensible to leave out the GPU. This blog post assumes that you will use a GPU for deep learning.
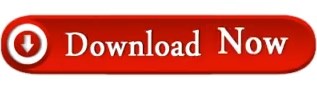